Thesis work
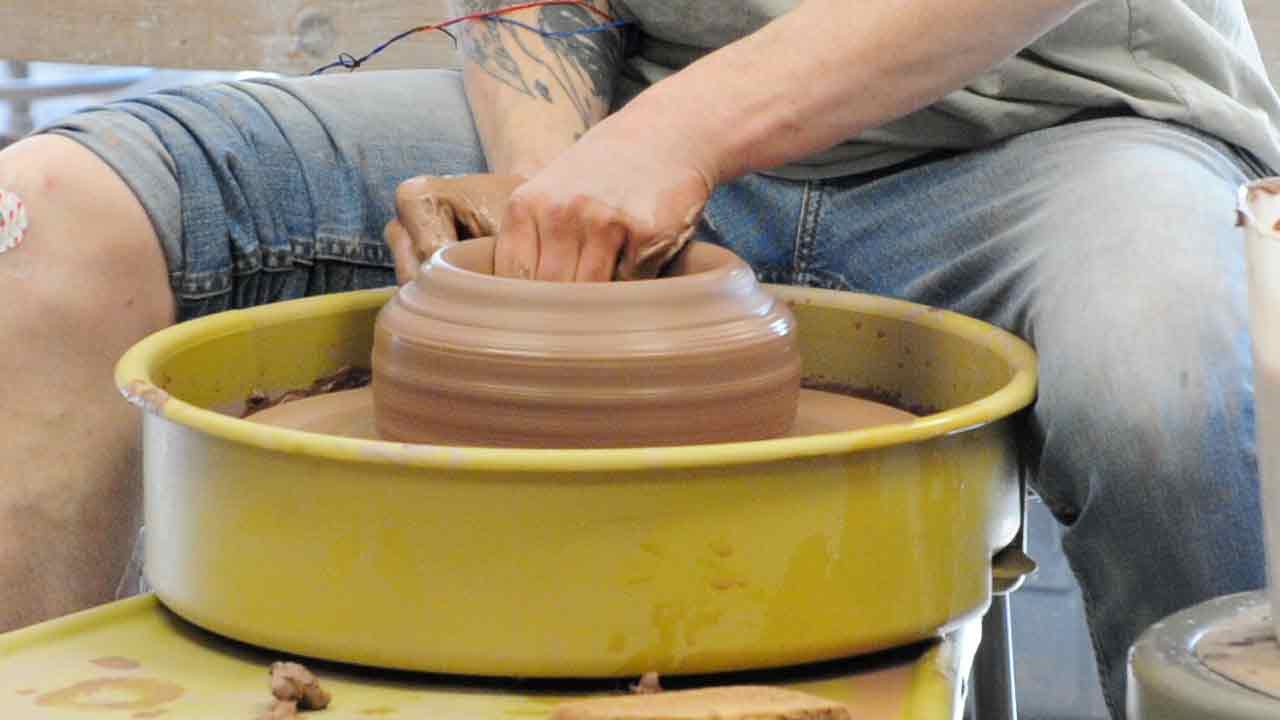
Imitating an instructor is traditionally at the center of the methods of learning motor skills in sports and crafts. Learners typically imitate based on visual clues about speed and timing, the positions and stances of different parts of their bodies, and other elements involved in mastering the skill. Watching someone else though, leaves little room for catching the subtleties of an instructor’s movement in real time or remembering them later. Our system overcomes this limitation by integrating real-time feedback that shows how the learner’s motion characteristics and those of the instructor correspond, providing a vivid, precise, and concrete way to compare and contrast movements. This makes it possible for learners to align their movements with those of the expert instructor without having to rely completely on memory.
We rely on our concepts of sensory motor learning that expand our perceptual framework when attempting to learn a new skill. For example, expert video gamers develop an extraordinary ability to extract information and extend their attention over a wider-than-normal spatial frame while experiencing no apparent decrease in attention. Notably, this integration process can account for the properties of external objects such as tools, which means optimal visuo-haptic integration even when tactile input comes through a hand-held tool.
Researchers such as Daniel M. Wolpert and team have highlighted motor learning processes and how they can be enhanced:
 
Learning can occur at different levels of the motor hierarchy. To understand how these changes are implemented in neurons, we need theories of the processes and representations through which learning is achieved. The processes of motor learning can be distinguished by the type of information that the motor system uses as a learning signal. Although different sensory modalities such as vision, proprioception and touch can all play an important part in motor learning, we focus here on the nature of the information, independent of modality, that is used during learning.
 
EMG-based feedback learning systems have been used for a multitude of skill-learning tasks such as basketball dribbling training, taking advantage of the differences in sharpness and timing between EMG signals from a novice and an expert. This use of EMG-based feedback was applied to the craft of pottery-making and successfully decreased the amount of time it took for a beginner to learn a simple task like centering the clay on a pottery wheel.
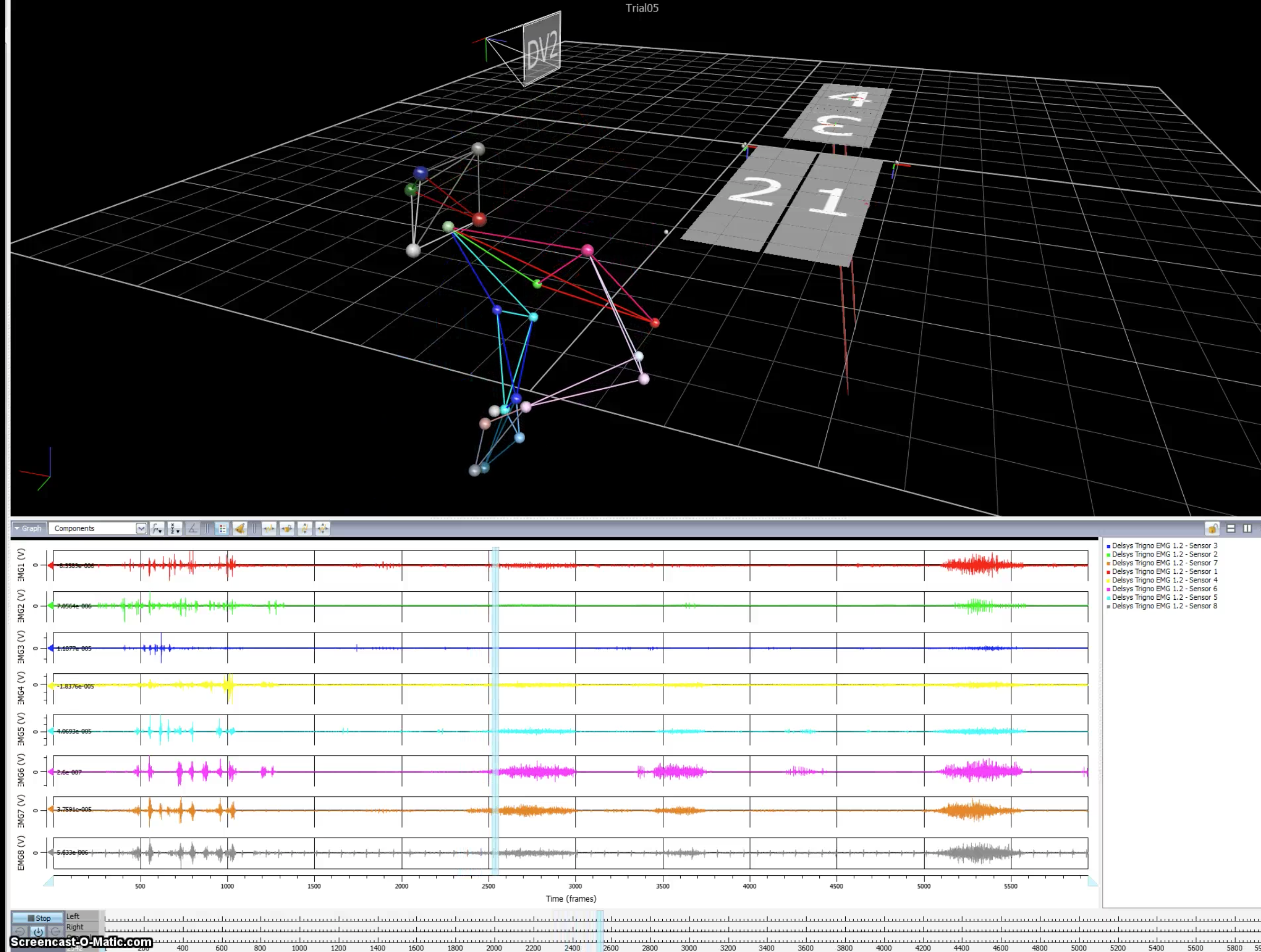
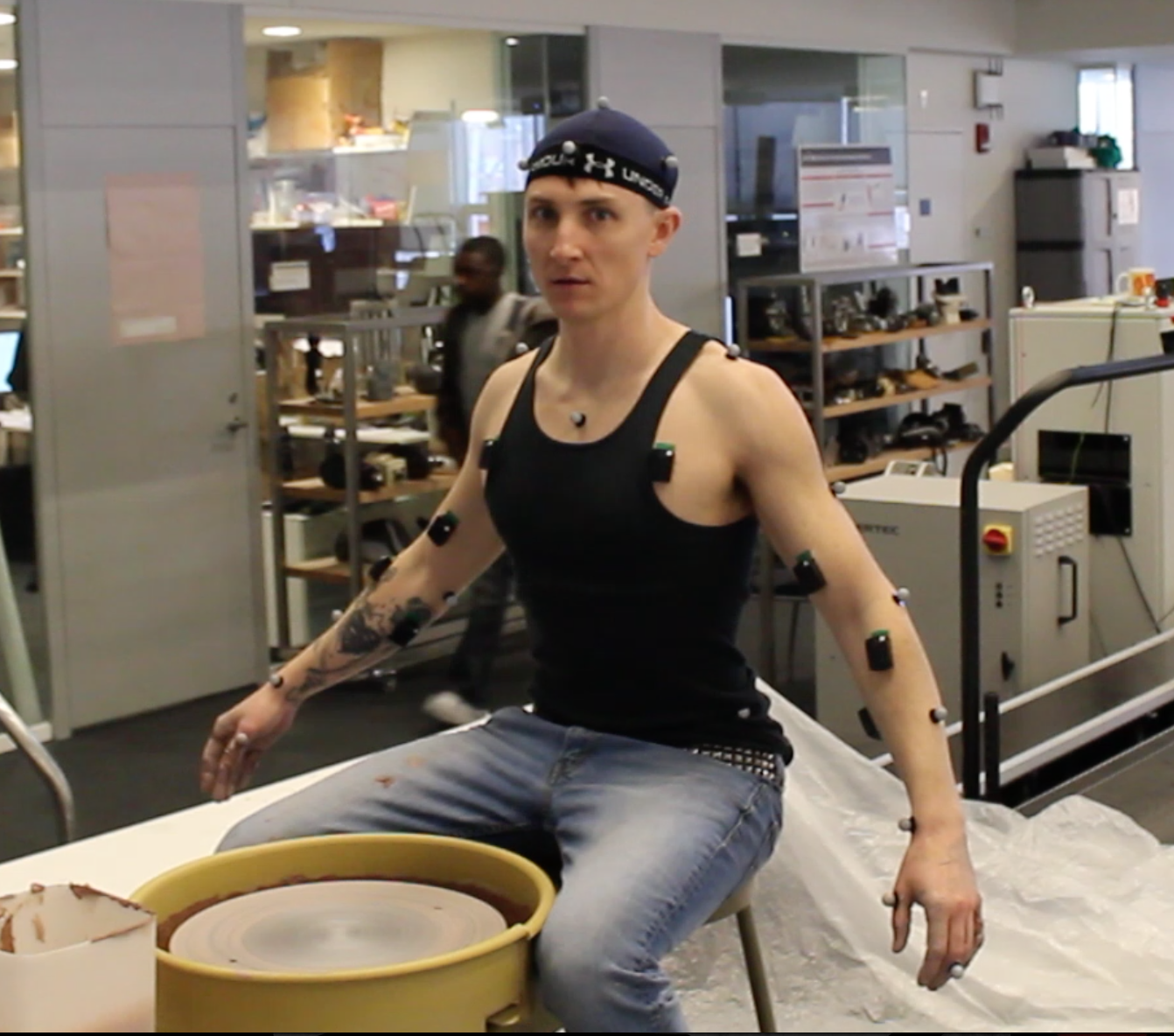
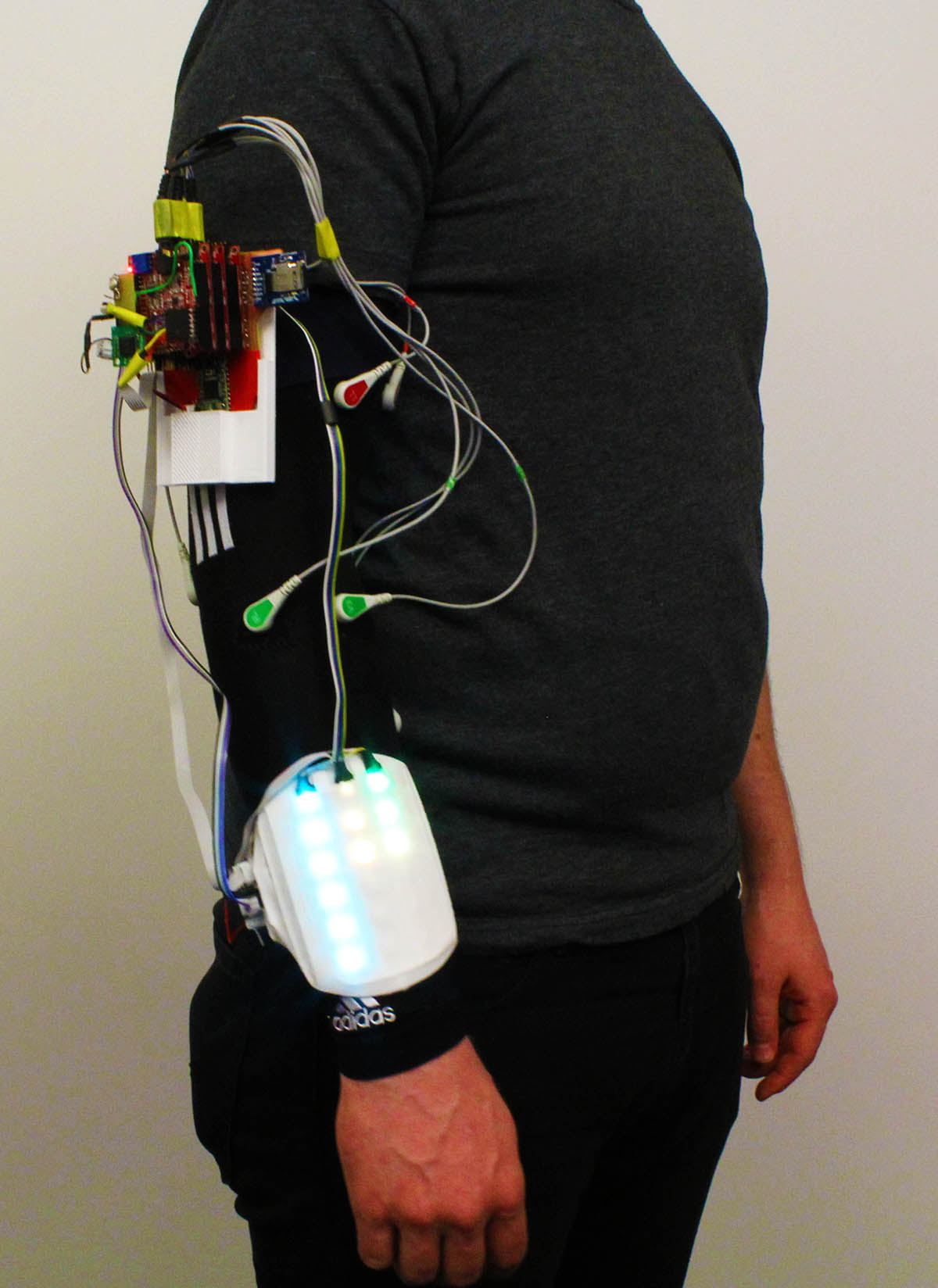
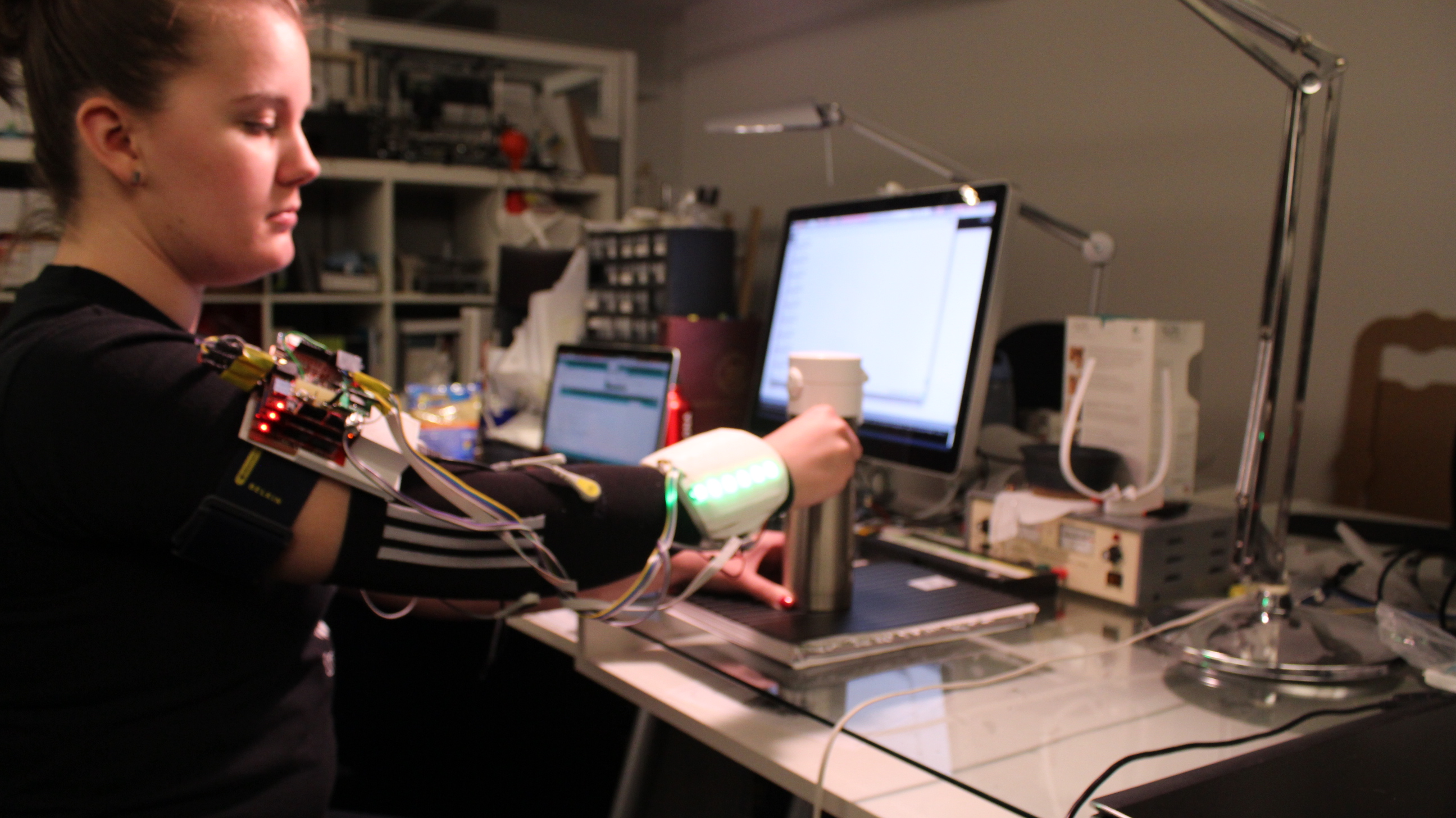